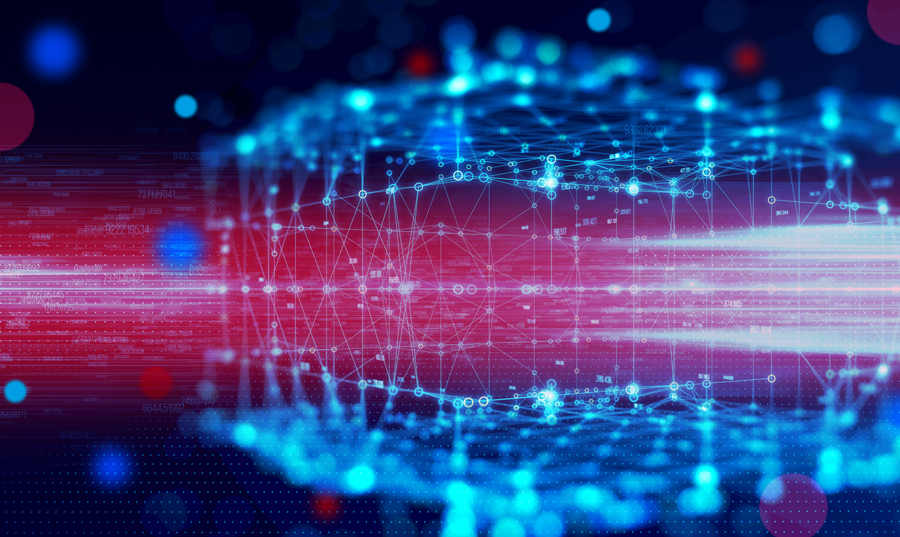
In recent months, I have discussed various use cases in which MediaKind is applying artificial intelligence (AI). AI is an incredibly fast-moving research topic, and we see it as a key focus area in the way we improve bandwidth efficiency and the energy footprint of our encoders – an essential element, particularly in the context of this fortnight. As a company, we are committed to environmental protection and reducing our environmental footprint by complying with all regulatory requirements, preventing pollution, and minimizing our impact to air, land, water, and energy consumption across the life cycle of our products and services.
But we are also focused on quality, which is why we are equally committed to providing products and services to our customers that meet or exceed their requirements. And that’s why we are constantly investigating new disruptive algorithms and techniques that can add value to our portfolio and state-of-the-art products and technologies for our customers. AI enables our industry to analyze data trends more rapidly and efficiently, accelerating algorithmic design and specifications. It’s also proven highly efficient in automatically detecting anomalies and behavioral issues, significantly reducing development time by detecting potential problems earlier in the process.
The impact of AI in video encoding
Earlier this year, MediaKind was awarded a 2021 Technology and Engineering Emmy Award for AI/Optimization for Real-Time Video Compression, which was a fantastic recognition of our role in pioneering the use of AI in the media industry. This helped provide the framework for our new AI-based compression technology (ACT), enabling us to enhance the efficiency of our product portfolio and reduce the consumption of resources. ACT provides our encoders with a comprehensive understanding of the video input and its characteristics, enabling our customers to automatically fine-tune the encoder parameters and maximize the compression efficiency according to their available resources.
By intelligently adapting as content changes, the encoder can always use its processing power to achieve optimal compression efficiency by dynamically modifying how processing is applied. As a direct consequence, operators can run a larger number of channels in the same computational resource. Alternatively, they can use low performance and more cost-effective hardware to achieve an equivalent compression efficiency with reduced energy costs. The saved resources can alternatively be used to enable additional encoding tools (which would otherwise remain disabled) to meet density or operational constraints, delivering improved rate-distortion efficiency for an equivalent footprint.
Using AI for content detection
AI is enabling us to deliver better content analysis, and it’s an area that has been gaining momentum over the past few years. Achieving an autonomous understanding of video semantics and effectively detecting actions and objects in the video opens possibilities to add new functionalities and services with added value to our customers, both in the media processing chain and in generating new revenue streams. For example, content detection could target and tailor advertisements or perform automatic parental control over certain types of content. The method can also generate automatic highlights for sports, provide indexing for video searches, or automatically generate captions and audio descriptions for the visually impaired.
Using AI to detect places, actors or celebrities can also be a practical way to provide extra information and context to what the viewer is watching and make it a more enriching, exciting, and immersive experience. Such information may also be of particular interest to generate new content suggestions or to assess interest in specific subjects or content.
Optimizing through Machine Learning
We recently launched a new application paper titled ‘Improving Video Compression with AI: Using Machine Learning to infer Coding Unit splitting for HEVC.’ It looks at how we can extend the use of Machine Learning techniques beyond previous methods and how a new generation of AI-powered encoders are helping to raise the bar on codec efficiency for the entire encoder industry.
But different ML approaches can address other areas, and one area that MediaKind is benefiting from is metric forecasting. As we look to elevate our team’s service standards, we have had to look for new ways to handle how we scale our operations, and with it, how we monitor and manage a multitude of metrics across a series of different dashboards.
We have been using Grafana Cloud to address this, enabling us to observe and ensure that our systems are consistently up and running. We initially used Grafana Machine Learning as part of a beta tester program and have since leveraged it to train machine learning models to rapidly identify network packet loss as the root cause of downstream video errors. The model has also learned the unique characteristics of an individual channel and can alert users to unusual activity.
In the second part of this blog, my colleague Richard Chin will be discussing the impact of Grafana Machine Learning metrics. They will also be addressing how our engineering teams use these key metrics to troubleshoot client challenges and meet service guarantees. Look out for that video in the coming weeks.